I mentioned that I was 1. making a gif and 2. working on the particle-based fluids for my CS5643 class. Well, here it is.
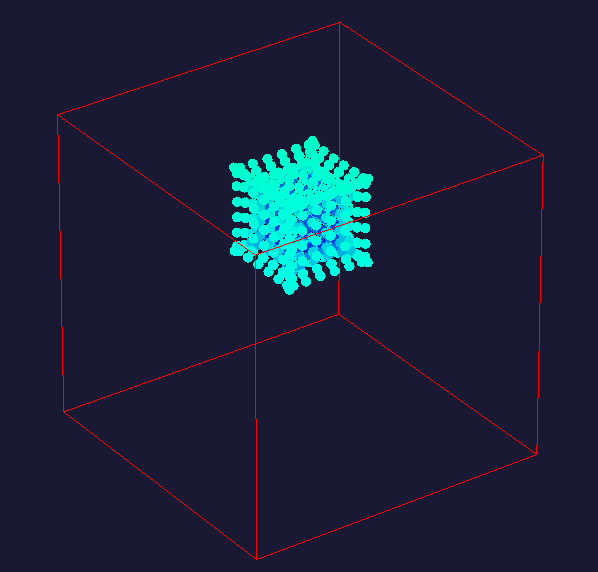
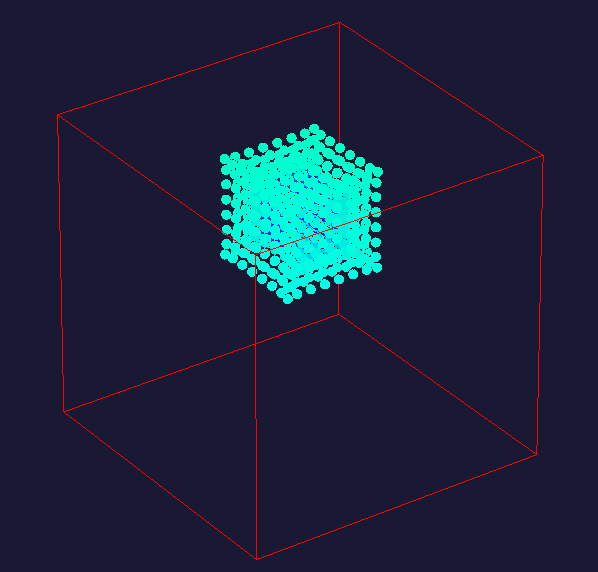
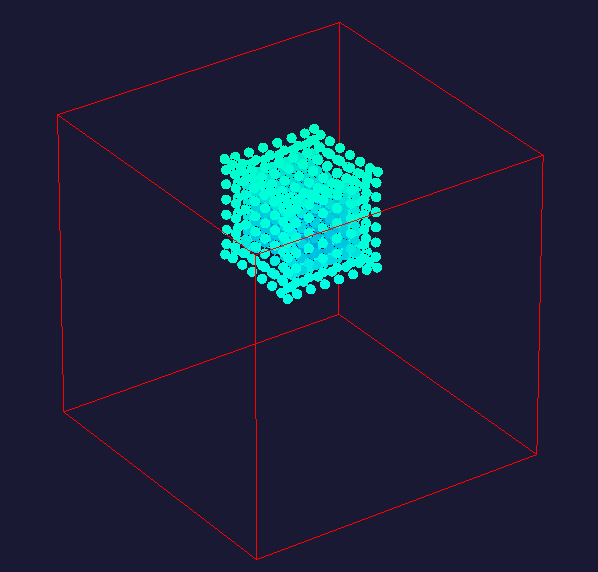
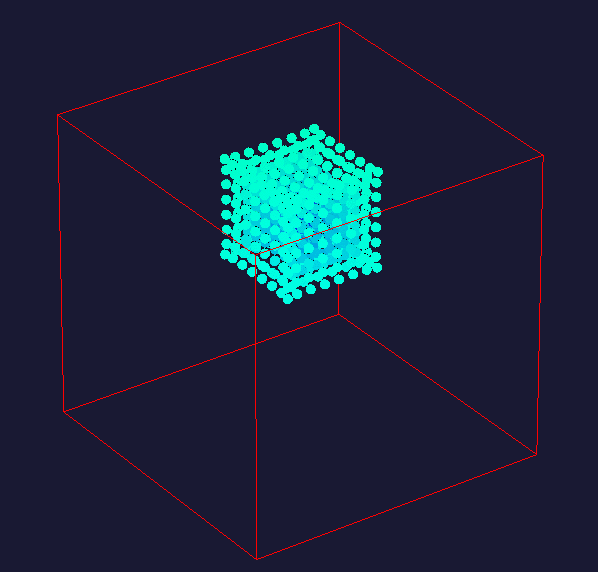
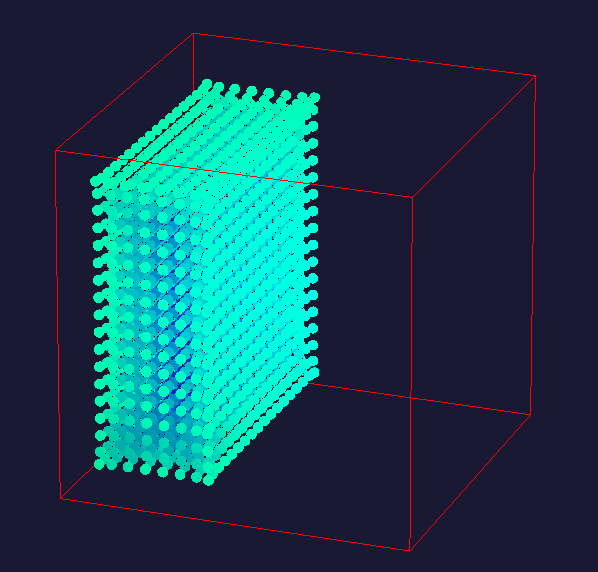
Take 4 at a stable blog
Stuff about math and programming. Nerdy shit.
I’ve been working on a few things:
Hopefully, what ends up happening is something like the following, except less pretty.
Turns out it just was infected gum.
-_- ugh I hate my life.
MY WISDOM TOOTH IS COMING OUT AND IT IS SO ANNOYING. COMBINE THAT WITH CANKER SORES AND A SLIGHT SORE THROAT, MY DAMN MOUTH IS SILLY.
Check out that subreddit:
I’m doing some programming work for Professor Bindel in the CS department, and it’s pretty novel stuff to me. I figured if I write out what I know about the original method which he is trying to improve on, it’ll help me with the overall picture. Here it is:
The entire premise of GMRES (generalized minimal residual method) is to solve the system of equations like where
is a matrices and
are vectors with appropriate dimensions. First though, we need some additional machinery before we can cover the main method (don’t worry, the machinery does most of the work).
First some definitions and an overview before nitty-gritty: a Krylov subspace is a magic thing which is quite simple to think about. It’s defined as . It’s really nothing but a series of vectors which we get from multiplying
with
lots of times.
What GMRES aims to do is to minimize the residual ( where
).
I’m going to assume that you seen what the Gram-Schmidt method is; if you don’t recall, it’s the algorithm where given a set of vectors that span a space, you return an orthonormal basis for the subspace which is spans. The Arnoldi iterations are something extremely similar to that, except it’s got a few caveats.
Imagine that we already have a bunch of the vectors from the Krylov subspace from above, but we really can’t work with them. They might be very poorly conditioned in that they’re basically pointing to the same direction (it resembles the power iterations). We basically apply GS on those Krylov vectors to obtain an orthonormal basis. Our end goal is to obtain an expression of the form where
was from the matrix defined in our problem and
matrices are the Krylov subspace vectors combined into a matrix. The
is called a upper Hessenberg matrix (i.e. an upper triangular matrix with the first subdiagonal filled in).
The algorithm is as follows (shamelessly copied from wiki):
What does it mean? It’s just like (modified) GS! For each additional vector from the Krylov subspace, you take out the stuff that are orthogonal to vectors already processed and store it in the matrix. Sounds simple but looks rough…
Now we can actually get to the method (which is simple if you’re still reading). We try to minimize the residual (in the Euclidean norm for those wondering), for
(i.e. the kth Krylov subspace). We can rewrite that as
as from our discussion of the Arnoldi iterations above,
is the matrices of the Krylov subspace.
Now, we perform some algebra…
The first equation comes from substitution, the second from using the Arnoldi iterations results, and the last step is kind of tricky. C in our equation is the norm of the projection of b onto the orthogonal complement (i.e. the subspace complement to the space) to the Krylov subspace. Think of this as saying the Pythagorean theorem on the part that lies within the span, and those that lies complement to it.
Finally,
The last bit is just the observation that the first column of V_{k+1}[\latex] is b, normalized to unit length. So
V_{k+1}^Tb=\|b\|e_1[\latex].
If you notice the Arnoldi iterations, you have more and more vectors to loop over as the Krylov subspace gets larger… and you have to store all those vectors too! This is why people consistently use GMRES with restarts, where they erase all the previous iterations, and use reconstruct the Krylov subspace from the current, closest solution.
The problem with this, is there are situations where convergence to the solution actually depends on the restart! If you don’t choose a good restart value, convergence to the solution might not occur. That’s bad.
Another way to deal with this is to demand the matrix of vectors by well conditioned… but I don’t know much about this. And another way, is to use Chebychev polynomials to somehow do it (more on this later after I read the paper).
I finally decided to drop algebra, which puts me at 20.5 credit hours this semester. Honestly, it wasn’t a tough decision.
More and more, I see my pure math as an mental excursion to go on when one wants to find fascinating sights. But that’s all it is though, a mental treat as I don’t see myself becoming a pure mathematician.
Am I smart enough for pure math? Maybe.
Am I motivated enough for pure math? Maybe.
One does not pursue grad school when the answer to those questions are just maybe.
The past summer, I was at the REU in UMBC. The head of the math department guy preached about the future of mathematics is the intersection of mathematics, statistics and computing; I agree to a large extent.
What I ended up working on seemed to be computing, with more computing, with very little mathematical analysis. The system of differential equations that described pancreatic cells was far too advanced for me to do some significant analysis. Heck, that’s how I feel about most of pure mathematics: the interesting problems are always out of reach from what I know.
Before this past winter break, I found a cool book called “Risk and Reward” in the math library. It’s all about the, surprisingly deep, game of casino blackjack, from the strategies to the mathematics behind the analysis. In the analysis portion of the book, 90
If I ever do become a professor of some sorts, I want to be able to teach a class based on games like blackjack and poker. For projects of sorts, the task will be to calculate the probability of various conditions and to figure out the strategies from the math I provide in class. Maybe for a (optional) final, it’s to actually play the games that we studied in class…
As an exercise over the break, I took an existing program and added the functionality to “practice” the card counting went over the book. It’s based on this project which I butchered (honestly, I spent 2 days doing this… trying to understand someone else’s code is tough). To run the program, make sure Python is installed, and run Blackjack.py. Download
On the recent Modern Family episode, Claire was seen giving a lecture to the AP Calculus teacher on how many hours each student are expected to spend each night on school work (totally off for the record…).
Strangely enough there was the word “Schrodinger’s” on the board. Never in AP calculus did I
To be honest, I’m not entirely sure of the derivation to that equation…
Still, they did get something right! The theorem to the far left seems to be Rolle’s Theorem!
Two real numbers x and y are chosen at random in the interval (0, 1) with respect to the uniform distribution. What is the probability that the closest integer to x/y is even? Express your answer in terms of pi.
If one were to draw the area where x/y is even, it’s pretty clear from the get-go that we will have something like the following:
I looked at the problem from the viewpoint of lines (x/y = 1/2, x/y = 3/2, …). This results in the following series from summing the area of the triangles (don’t forget to divide by 2):
Luckily, the series at the end is basically from the arctan series expansion. Thus the answer is
.